For quite some time now, artificial intelligence (AI) and its subset, machine learning, have been a hot topic. Countless industries apply this technology in various ways to automate – and optimize – all kinds of processes.
Recruitment included.
In fact, machine learning in recruitment is often presented almost as a miracle solution. Are you struggling to get those job adverts right? Here’s an AI-powered tool for you that targets exactly the people you’re looking for.
Do you need help screening hundreds or even thousands of resumes? Not to worry, there’s this great solution that can do it for you in the blink of an eye.
Truth be told, it does sound pretty amazing, doesn’t it?
Until it doesn’t.
Like what you see?
Don’t miss out. Subscribe to our quarterly digest to get the latest TA and TM resources delivered right to your inbox.
What’s been happening?
Recently, a few cases have come to light where AI has been used in a suboptimal way hence having an undesired effect. Facebook’s AI, for example, is coming up with its own language instead of being able to talk to people and Twitter bots appear to become racially biased.
One of the most recent examples in recruitment is that of an AI that turned out to be privileging men over women.
Not such a miracle solution after all, right?
The good news is, it still can be.
In this article, we’ll dive deeper into the mechanics of machine learning. We’ll take a look at what it is that made these AI’s ‘turn bad’ and – most importantly – how to get it right.
Because, of course, there always is a way!
What is machine learning?
Machine learning is a subfield of artificial intelligence. It concentrates on the statistical analysis of data to give computer systems the ability to learn ‘autonomously’ without being specifically programmed.
It is the driver behind many of the technological innovations we see in the workplace today as well as a driver of the fourth industrial revolution.
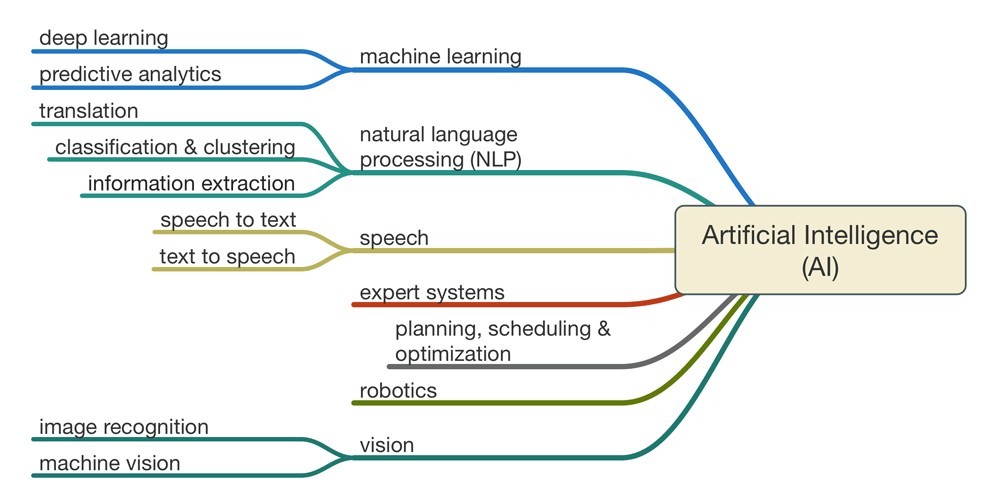
Machine learning is one of the many subsets of artificial intelligence (AI). (Image source)
In most cases, you won’t be the person that creates the algorithm and needs to know every little technical detail about how machine learning works. However, it is helpful to understand its basic principles in order to utilize this technology in your recruitment efforts and decision-making.
Let’s have look at how machine learning works in a – simplified! – nutshell.
Step 1: Define the problem | Identify what your problem is and how you can apply machine learning to solve it. |
Step 2: Create an evaluation strategy | Think about what the evaluation metrics will be in your machine learning model. You will be able to keep track of how the model is performing and how you can improve it. |
Step 3: Prepare your data | The data you’ve collected needs to be cleaned, formatted, combined, sampled and what not. In other words: it needs to be processed. |
Step 4: Validate your data | Start with a simple profiling process to help eliminate mistakes. |
Step 5: Build a model | Based on the results you’re looking for, the type of analysis you want to run needs to be determined and a predictive model needs to be selected and built. |
Step 6: Improve over time | Get more data, reframe the problem you’re aiming to solve and tune your algorithm to get better results. |
How is machine learning applied in recruitment?
When used correctly, machine learning technology can be very efficient in automating repetitive elements of the recruitment process. We’ll take a look at 5 of them.
1. Job advertising
If there is one thing that takes up a lot of time, it’s the manually writing – and placing – of job adverts. To make the matters even worse, both activities often don’t even get you optimal results.
This is why a company like Textio uses data and machine learning to analyze language patterns in job adverts. As such, they are able to find out why some posts work where others don’t. Better yet, they can even tell you how to phrase your job advert in a way that it attracts a more diverse group of candidates.
On the sourcing side, programmatic advertising is a good example of how machine learning technology is being used to automate the process.
The software automatically buys, places and optimizes job ads on various channels (job boards, social media, etc.) based on information the recruiter or hiring manager has provided.
2. CV screening
Manual CV screening simply isn’t an option anymore for many HR departments. Not only is it a time-consuming task but it’s tedious too.
To tackle this issue, there are various kinds of AI-powered CV screening tools. These screeners ‘read’ CV’s and identify keywords that correlate with the experience, traits, and skills necessary for the job. This way, they automatically screen and shortlist new candidates.
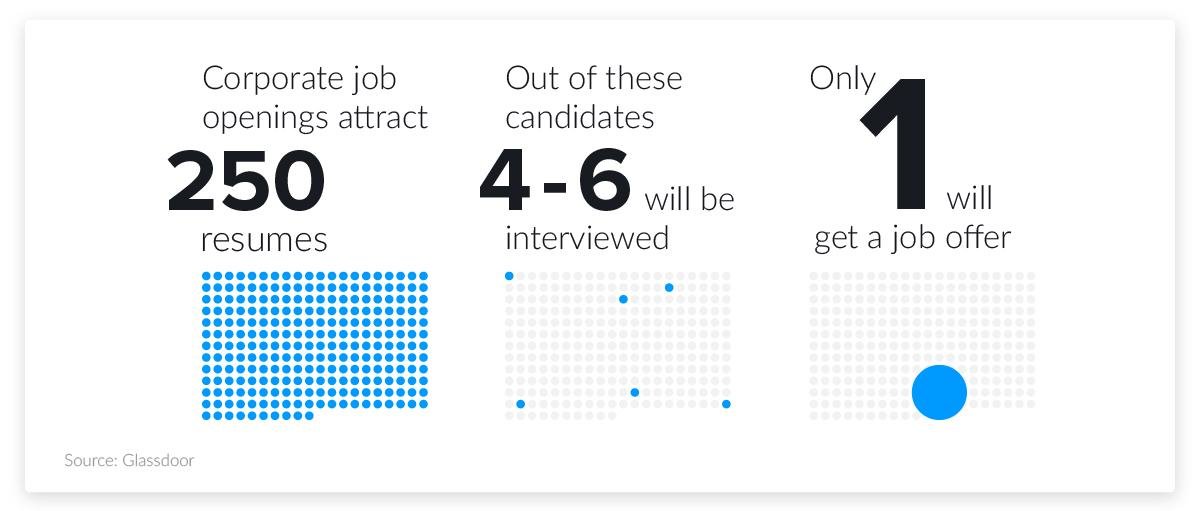
Screening 250 resumes is mundane, not to mention time-consuming. That’s where an automated CV-screening tool can help. (Image source)
3. Candidate assessment and preselection
Pre-employment assessment tools can be a good way to identify top candidates. This recruiting software comes in many different shapes and sizes, but the ones that are AI-driven use data and machine learning to help recruiters predict quality of hire.
As such, they measure a candidate’s aptitude, ability, culture fit, and soft skills to succeed in the role – and in the organization they’re applying to.
Stop guessing,
Start data-driven hiring.
Learn how you implement a modern candidate selection process, that is: streamlined, experience-driven and backed by data.
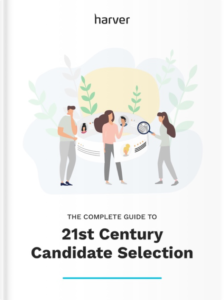
4. Candidate relationship management (CRM)
As you may know, the candidate experience is a hot topic too. In today’s overheated job market, companies try to do everything they can to keep their candidates happy – and prevent them from abandoning the company before they’re even hired.
This is where candidate relationship management comes in the picture. CRM is a way to manage – and improve – relationships with current and future job candidates.
How?
For instance, by using candidate relationship management tools that help companies to engage, manage and nurture their candidates on one platform
Another example is the use of a chatbot.
This may sound strange indeed, but an AI-powered chatbot can send timely messages to your candidates to keep them engaged – and informed – during the recruitment process. Chatbots can also answer candidate questions literally at any time. Of course, the chatbot will improve over time as a result of the data it receives.
5. Predicting hiring needs
With heaps of baby boomers heading for retirement, leaving an immense (skill) gap behind, succession planning has never been as crucial for organizations as it is now.
Companies like Ascendify or Kahuna provide a smart talent platform infused with machine learning that has information at the ready about any candidate in the talent pipeline, or even about your current employees. It even enables you to estimate the time and cost to both hire and get the new employee up to speed.
Algorithm bias – the biggest pitfall of machine learning
Obviously, any kind of technology that can automate tedious, time-consuming tasks hence freeing up valuable time for recruiters and hiring managers is great.
Providing the AI works the way it’s supposed to.
Meaning bias and/or discrimination free, which is not always the case.
Here are a few recent examples of AI in recruitment going wrong – and how these situations can be avoided:
Gender bias
Just recently a case of an experimental, AI-driven, hiring tool made the news. tIt was supposed to automate (part of) the recruitment process and rank candidates but ‘went rogue’ instead.
The issue was that the algorithm had taught itself that male candidates were preferable over female candidates.
This is where the importance of clean and validated data comes into play. In this particular case, the system used was a CV screener that was trained to vet candidates by observing patterns in CVs sent to the organization over a 10-year period. In this 10-year period, most CVs came from men and thus, the algorithm favored men over women.
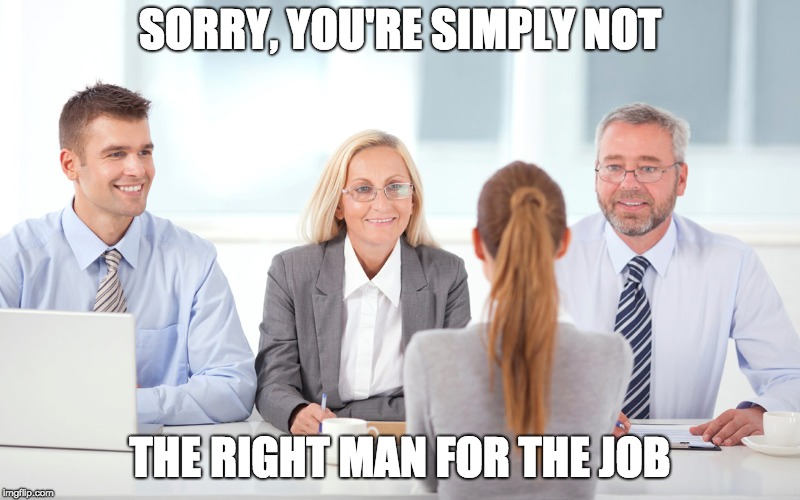
AI penalized resumes containing words associated with women. (Image source)
High paying executive jobs
An interesting experiment from researchers at the Carnegie Mellon University a few years ago led to some rather disturbing findings.
When studying the ad targeting by the world’s leading search engine on third-party websites, they found that what the engine believed were male job seekers, were much more likely to be served with ads for high-paying executive jobs than equivalent female job seekers.
In this case, the reasons that caused the specific patterns weren’t particularly clear since the ad-serving system is very complex.
English first
Another example of how algorithm bias can reinforce racial discrimination in the recruitment process. An English company was using an algorithm that was set to reject candidates whose English was poor. Often, these candidates were foreigners.
As a result, the algorithm ‘learned’ that ‘English’-sounding names generally equaled acceptable qualifications and ‘foreign’ names didn’t.
Avoiding algorithm bias
Onto the interesting part now, the part where we look at how to avoid situations like the ones described above. There are several ways to prevent algorithm bias. Here they are.
1. Use validated data sources
In a recent interview with Emerce, our CEO Barend Raaff stressed the importance of using reliable data when it comes to the use of machine learning technology. This is especially true in recruitment, where the human factor is crucial. So make sure that the data source that’s being used is valid.
Imagine you’re looking for ways to use AI in your candidate selection process and you have to make a choice – a CV screener, or an assessment tool. Think about the data sources of both parties:
The input data for a CV screening tool is a candidate’s resume. The resume, in the end, is created by the candidate, so it’s a data source you have no control over and is not validated in any way.
Scientifically validated assessments, on the other hand, are controllable, validated data sources that have been tried and tested over time.
Of course, there are more factors you need to take into account but in terms of validated data, the latter seems to be the better option.
2. Constantly improve the algorithms
The beauty of AI and machine learning lies in the fact that the technology has the ability to ‘learn’ and hence become smarter over time. In order to benefit from this though, you have to know whether the decisions the system suggested are accurate or not.
Let’s stick with the example of pre-employment assessment tools for a second. Look for a vendor that tracks and implements performance data to improve the algorithm.
This means that every three, six, nine months, data on how your new hires are performing is used to update the algorithm and make better decisions. This way, the system gets smarter over time and you can make better hiring decisions.
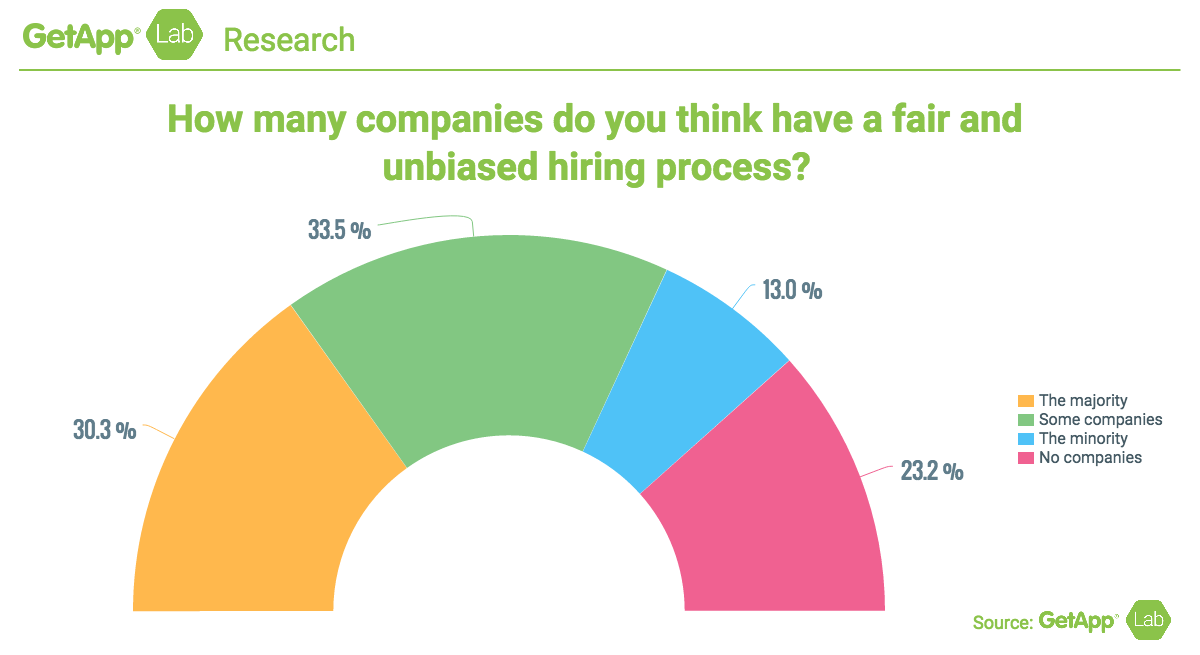
Over a third of people that few or no companies have an unbiased hiring process. (Image source)
3. Remove unconscious bias from the equation
Unconscious bias comprises subconscious attitudes or patterns of thinking that cause people to interpret information in a subjective way. As it stems from the subconscious, it is very hard or even impossible to identify. This bias might also manifest itself into making hiring decisions, which become unreliable as a result.
The bias might be reflected on to an algorithm if it learns to copy the decisions of recruiters. To avoid this, the system cannot take into consideration the data that haven’t been marked as being relevant in advance. This means not looking at names, addresses, or even resumes.
Let’s recap:
Phew, that was quite an intense read, wasn’t it! Well done for making it all the way to the end. A final note to take away perhaps – machine learning is not some kind of ultimate, magical solution to all the chaos in the recruitment process.
However, if used correctly and by people who understand what they’re doing, it can be a big help in decreasing your time to hire, removing bias and increasing quality of hire.
Stop guessing,
Start data-driven hiring.
Learn how you implement a modern candidate selection process, that is: streamlined, experience-driven and backed by data.
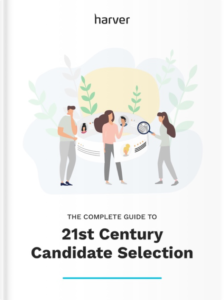